Cut support costs with a window into contact center dark data
Key points to unlocking your customer service dark data:
- Contact center is the heart of your customer conversations where brand loyalty relationships can be built or broken costing $9.7 million/year due to bad data
- True cost of bad data: customer churn, agent attrition, & operational efficiency & time
- Simplify the complexity to understanding your conversational data customer service topics and reasons to improve customer service
What does customer service have to do with dark data?
- Language data exists in vast quantities across the digital world but hasn’t traditionally been a source of insight
- Customer service unstructured dark data examples: call-center transcripts, text messages, survey responses, customer reviews, emails, documents, and more
- The challenge: bringing order to the chaos of dark data problems—too much data, inaccurate data, & poor data quality
- Example of challenge: manual review of transcript data that costs you time and resources
- Example of solution: simplified and automated process to understand your contact center conversations and customer service topics across channels with your agents and bots
- The result: data sources become functional & insightful to improve customer service vs. dead weight
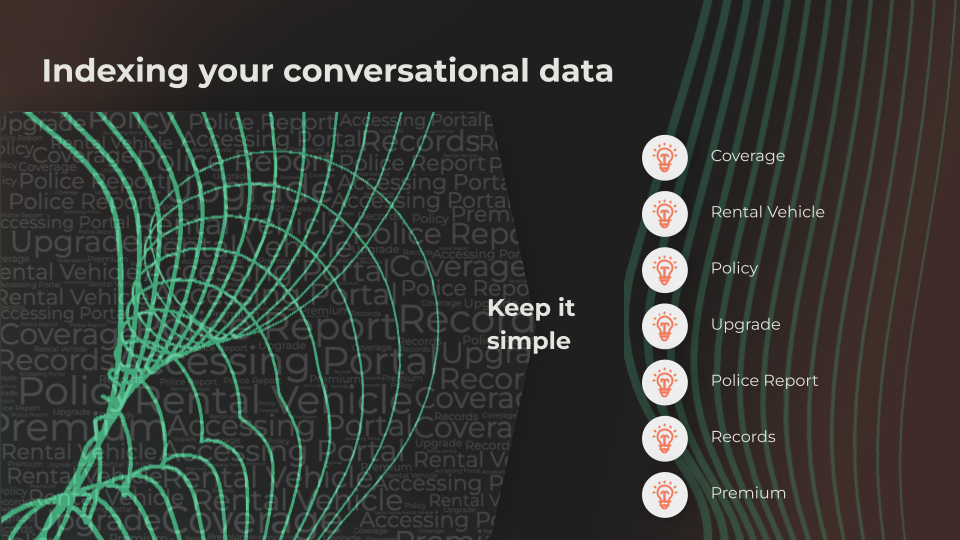
Simplifying Data Complexity
Unstructured dark data source example chat transcript
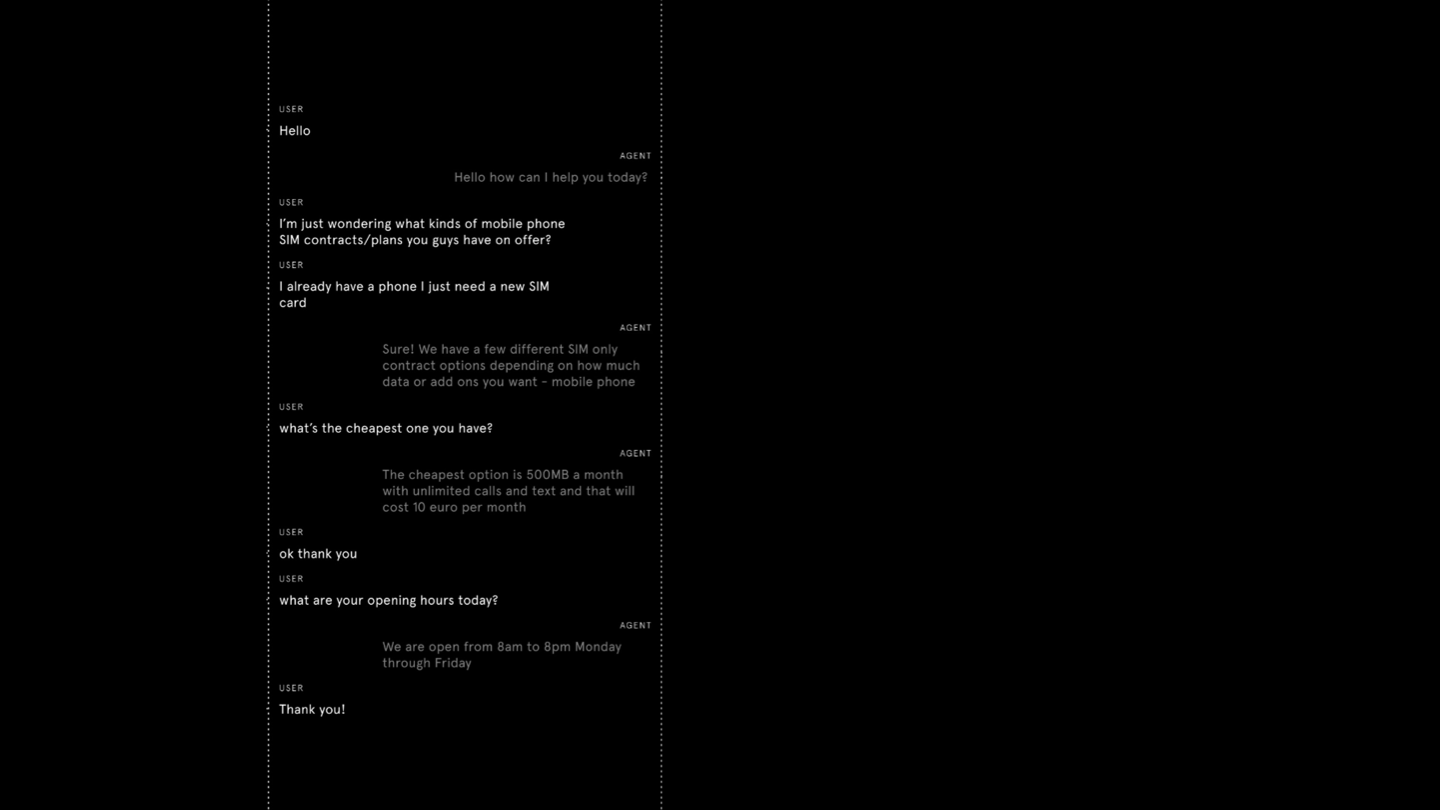
Chat transcript -> customer service topics
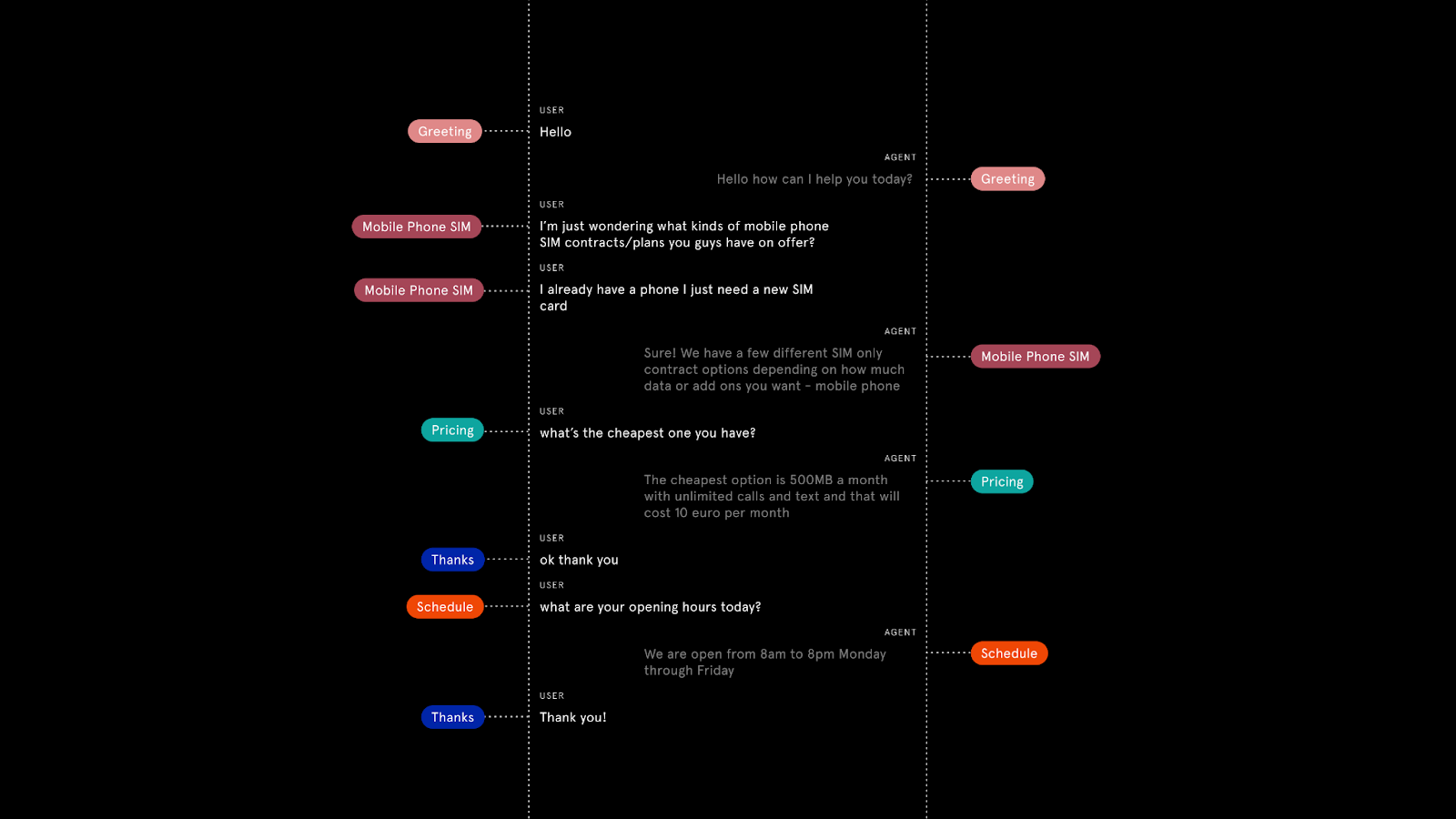
Chat transcripts -> customer service topics -> reason
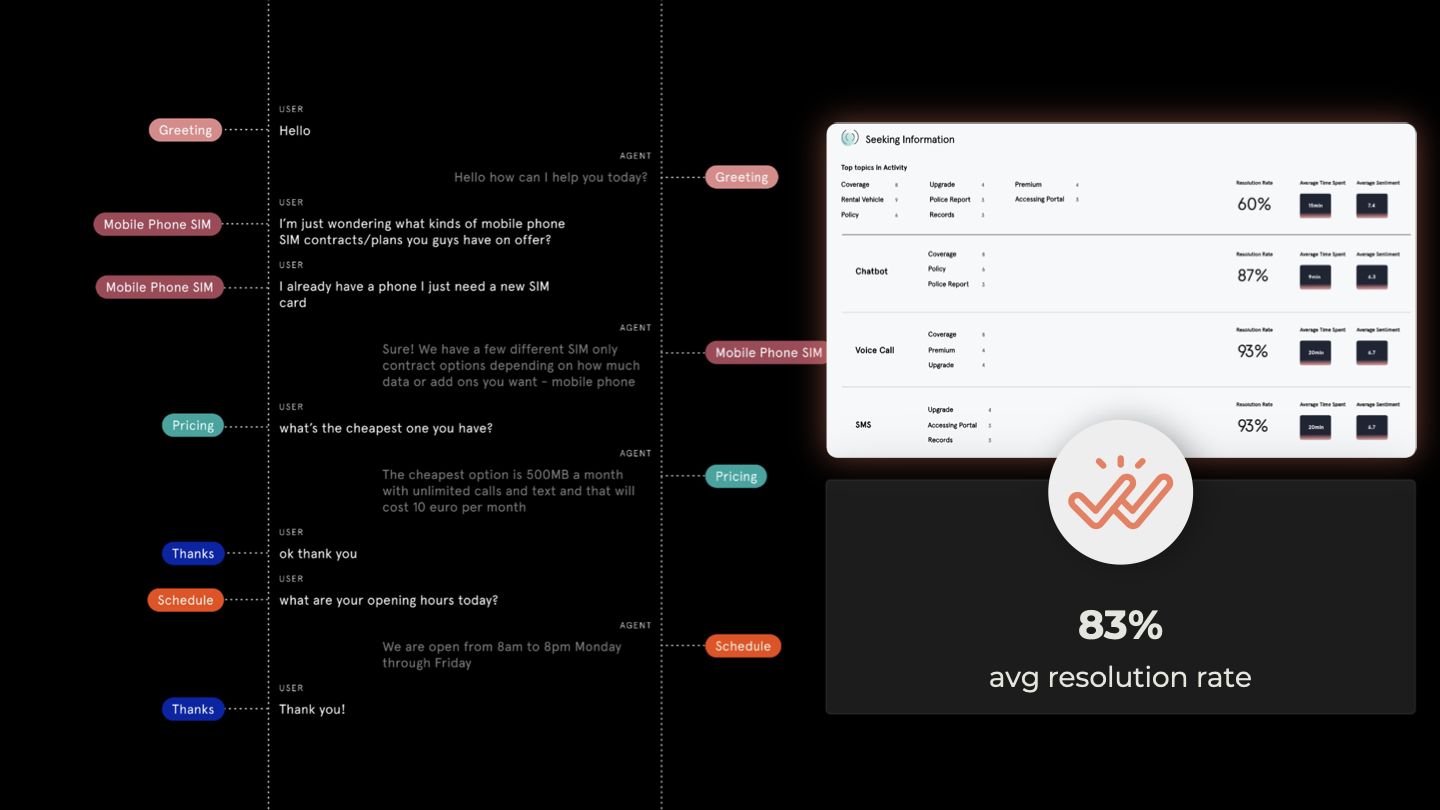
Balancing NLP & Prompt Engineering
Constraints of solely relying on prompt engineering are cost & precision:
- Bound by API constraints (e.g. limited adjustable parameters, guardrails imposed by creators, compute time - e.g. 20 seconds per prompt)
- Prompt engineering isn't a solved problem and may not be fully optimizable in principle due to the natural language format
- Traditional NLP methods offer precise control over pipeline/output + are more optimal in some cases (e.g. topic clustering for vectors)
Harmonizing prompt engineering + traditional NLP methods based on what is optimal. Example workflow:
- Vectorize language data
- Cluster into topics
- Feed topic data into prompts for label creation
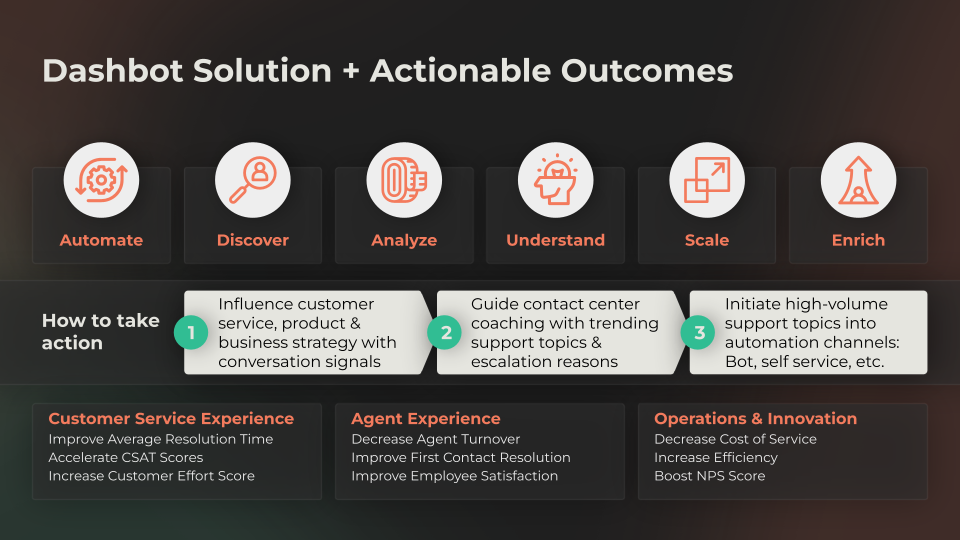
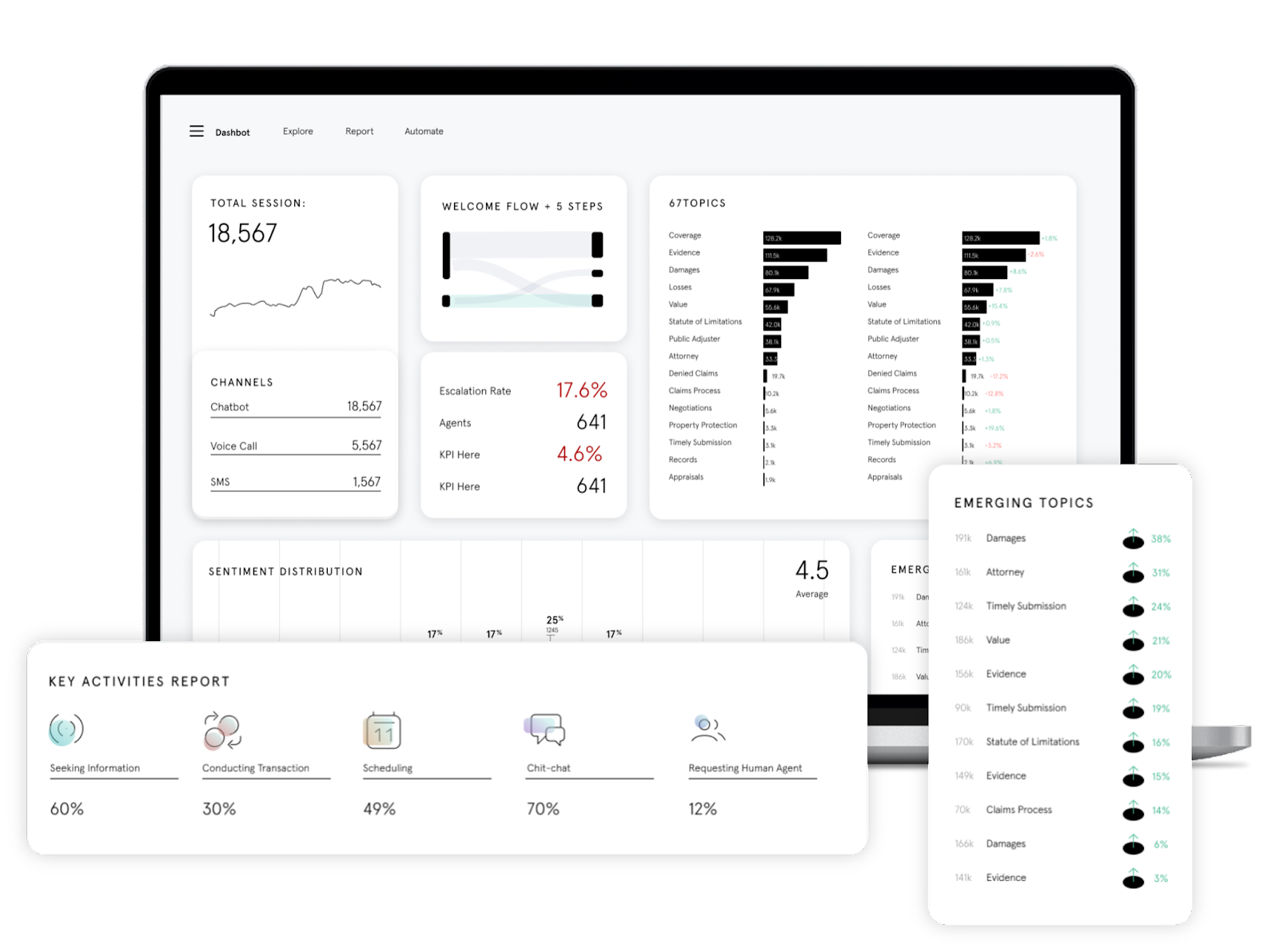